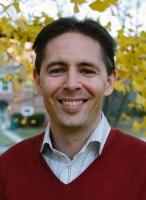
Information is one of the most valuable commodities in the world, and systems often face the task of trying to manage large datasets while uncovering the most relevant information. This is true in the case of biological neural systems trying to use their senses to understand the environment. It is also true for engineered systems, including neuroengineering applications where radical new tools are emerging to observe and manipulate complex neural systems. Members of the SIPLab perform research aimed at understanding biological information processing systems as well as developing novel algorithms for large-scale data science. The research areas in the lab include foundations in neuroengineering, machine learning and signal processing. The detailed work including practical algorithm development, modeling, and theoretical analysis. Application areas are always evolving, but currently include:
- computational and theoretical neuroengineering in several systems (e.g., vision, audition, somatosensation), paradigms (e.g., artificial stimulation, electrophysiology with natural scenes) and tasks (closed-loop control);
- dimensionality reduction, manifold learning and dynamic filtering;
- imaging and remote sensing (including hyperspectral imagery);
- computer vision and human-computer interaction; and
- novel neuromimetic computing approaches.